Algorithms at the forefront: Safeguarding our digital privacy
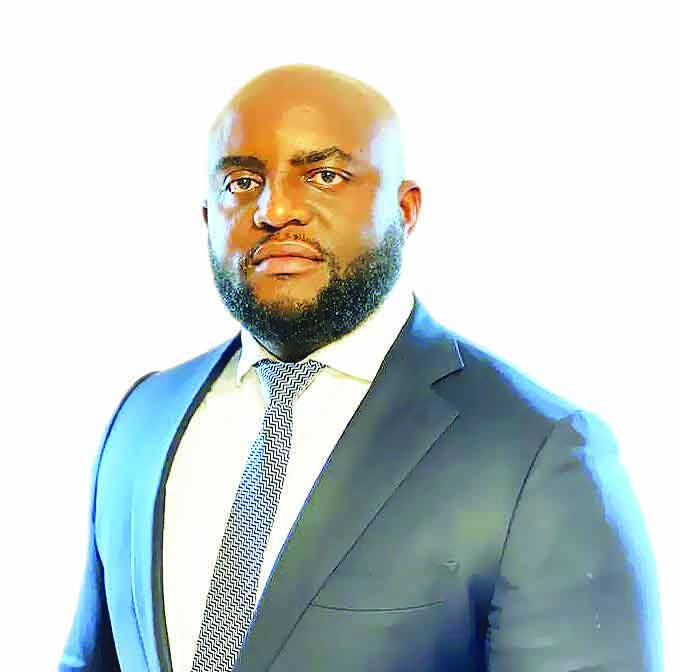
Michael Irene is a data and information governance practitioner based in London, United Kingdom. He is also a Fellow of Higher Education Academy, UK, and can be reached via moshoke@yahoo.com; twitter: @moshoke
February 21, 2024469 views0 comments
As a Data Privacy and Protection Specialist, I have observed the delicate balance between data utility and privacy within the digital ecosystem. Our daily digital interactions, be they voice commands to a virtual assistant, fitness tracking on a smartwatch, or route optimisation on our smartphones, are underpinned by algorithms. These interactions leave behind a trail of data. My role has evolved to leverage the prowess of algorithms and machine learning to shield these data points, aiming to preempt privacy threats before they even surface.
Imagine your routine morning: you interact with various smart devices, each collecting data to personalise your experience. Yet, as these algorithms assimilate information, the shadow of privacy concerns looms larger. The challenge I face daily is how to employ machine learning to fortify privacy rather than erode it.
Machine learning is inherently data-hungry; the more it consumes, the more intelligent it becomes. However, this voracity presents a conundrum in the context of privacy. My work involves constructing models that bolster privacy defences — for instance, algorithms that pinpoint abnormal data access patterns, flagging potential breaches proactively. I’ve developed systems using machine learning to differentiate between harmless and potentially intrusive data access patterns, allowing for early intervention.
Take, for example, the smart thermostats in our residences. They adapt to our schedules and preferences, optimising comfort and energy efficiency. Nevertheless, this data could inadvertently disclose when we are absent, entailing a privacy risk. In response, I’ve seen the emergence of algorithms designed to obscure these usage patterns, thereby preserving comfort without surrendering privacy. These algorithms cleverly distort data to remain functional for energy conservation while obscuring personal routines.
Consider the recommendation engines on e-commerce platforms. They anticipate our next purchase based on our browsing and buying history. This convenience simultaneously constructs a detailed profile of our consumer behaviour. To tackle this, I’ve engaged in projects that employ differential privacy, where algorithms introduce a deliberate degree of uncertainty into the data analysis process. Consequently, product suggestions stay pertinent, but the precise nature of an individual’s shopping habits remains hidden.
Looking forward, the synergy of machine learning and privacy is set to become increasingly pivotal. The advent of Federated Learning, where machine learning models are trained across numerous decentralised devices, each holding data samples, provides a glimpse into this future. This method allows algorithms to learn without central access to personal data, a concept I regard as a potential game-changer in privacy preservation.
During my tenure, I’ve also noted the burgeoning trend of Synthetic Data Generation — algorithms crafting entirely artificial datasets that replicate the statistical properties of genuine data. This innovation enables the training of sophisticated models without risking actual personal data exposure. For example, medical diagnostic AI could be trained using synthetic patient data that never corresponded to real individuals, thereby nullifying privacy risks while still advancing medical technology.
However, future-proofing data privacy transcends technical solutions. It necessitates a cultural paradigm shift. Just as I advocate for ‘privacy by design’ in technological development, I champion a societal norm of ‘privacy by default’. It’s imperative to construct secure systems; yet, educating and empowering individuals to manage their digital footprints is equally crucial. From teaching children about digital privacy to informing adults about the ramifications of their online activities, the cultural dimension is as critical as the algorithms we devise.
Regulatory frameworks such as the GDPR have been instrumental, but as we advance, they too must evolve in tandem with technological advancements. I am actively involved in dialogues intended to influence future legislation that is resilient yet adaptable enough to keep pace with swift innovation. The equilibrium between data utility and privacy is continually shifting, and our legislation must be agile to remain effective.
In summation, the destiny of data privacy is influenced by the very algorithms that pose a threat to it. In my professional capacity, I stand at the vanguard of this conflict, employing machine learning as both a protector and a strategist. By moulding the algorithms and the ethos surrounding them, I endeavour to ensure that our digital progression does not outstrip our entitlement to privacy. It’s a complex and ever-evolving domain, but one where I believe the right amalgamation of technology, education, and regulatory oversight can secure a future where privacy is not a luxury, but an inherent right.
business a.m. commits to publishing a diversity of views, opinions and comments. It, therefore, welcomes your reaction to this and any of our articles via email: comment@businessamlive.com