Data minimisation in machine learning dynamics
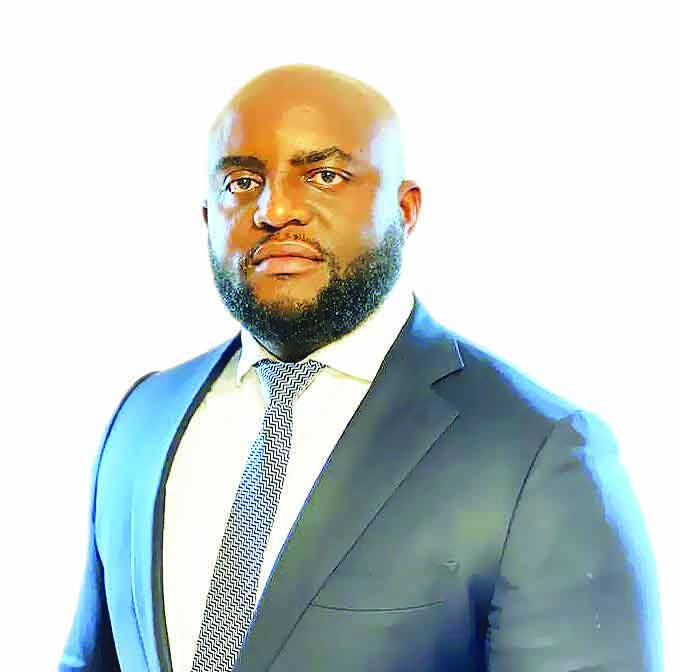
Michael Irene is a data and information governance practitioner based in London, United Kingdom. He is also a Fellow of Higher Education Academy, UK, and can be reached via moshoke@yahoo.com; twitter: @moshoke
January 29, 2024702 views0 comments
In the realm of data protection, the interplay between machine learning and the principle of data minimisation takes centre stage, shaping the landscape of privacy and innovation. Data minimisation, a fundamental tenet of responsible data handling, advocates for the collection and processing of only the necessary information required for a specific purpose. As technology, particularly machine learning, continues to advance, navigating this delicate balance becomes both a challenge and an opportunity in the digital age.
Machine learning algorithms thrive on data — large volumes of it. These algorithms learn from patterns, making predictions or decisions based on the information fed into them. However, the ethical use of these powerful tools hinges on a nuanced approach to data minimisation. Striking the right balance involves extracting meaningful insights while respecting individual privacy rights and adhering to stringent data protection regulations.
Consider the scenario of a retail giant employing machine learning to enhance customer experience. By analysing purchase histories and browsing patterns, the algorithm can recommend personalised products, improving user satisfaction and boosting sales. However, the ethical tightrope comes into play when deciding how much customer data is necessary for effective personalisation.
Data minimisation, in this context, entails honing in on pertinent details — purchase preferences, for instance — while excluding sensitive information unrelated to the task at hand. An individual’s shopping habits can be deciphered without delving into their private life details, aligning with the spirit of data minimisation.
The financial sector also grapples with this delicate dance between machine learning and data minimisation. Banks, armed with predictive algorithms, seek to assess creditworthiness efficiently. By analysing transaction histories and financial behaviours, these algorithms can make rapid and informed lending decisions. However, the challenge is to streamline the information collection process, ensuring only essential data is utilised without compromising the accuracy of risk assessments.
One compelling example comes from healthcare, an arena where the potential of machine learning is vast. Medical researchers harness algorithms to analyse vast datasets, identifying patterns that can lead to breakthroughs in disease diagnosis and treatment. Here, data minimisation involves extracting relevant health indicators while safeguarding patients’ personal information.
Consider a scenario where a machine learning model assists in diagnosing a rare medical condition. By focusing on specific symptoms and genetic markers, the model can contribute to precise diagnostics without requiring an exhaustive personal medical history. In adhering to data minimisation principles, the healthcare sector navigates the ethical terrain of leveraging technology for societal benefit while respecting individual privacy.
The interplay between machine learning and data minimisation extends into the realms of artificial intelligence-driven hiring processes. Companies increasingly deploy algorithms to sift through job applications, identifying potential candidates based on predefined criteria. Here, the challenge is to strike a balance between efficient recruitment processes and the responsible use of personal information.
Imagine a scenario where a machine learning algorithm screens job applicants based on relevant skills and qualifications while omitting factors such as age, gender, or ethnicity. This exemplifies the application of data minimisation in recruitment, ensuring fair and unbiased selection processes.
As the regulatory landscape evolves, particularly with the General Data Protection Regulation (GDPR) in the European Union, companies must grapple with the legal implications of their data handling practices. GDPR explicitly underscores the importance of data minimisation, urging organisations to limit data processing to what is strictly necessary for the intended purpose.
In essence, the interplay between machine learning and data minimisation is a delicate dance between technological advancement and ethical considerations. Striking the right chord requires a commitment to responsible data stewardship — leveraging the power of machine learning for innovation while respecting individual privacy rights. As we navigate this complex landscape, the synergy between these two forces will shape the future of data-driven industries, defining a path that balances progress with ethical integrity.
- business a.m. commits to publishing a diversity of views, opinions and comments. It, therefore, welcomes your reaction to this and any of our articles via email: comment@businessamlive.com